Table of Contents:-
- Data Processing Meaning
- Importance of Data Processing
- Data Analysis Meaning
- Objectives of Data Analysis
- Types of Data Analysis
Data Processing Meaning
After collecting the data, it needs to undergo processing and reduction to make it suitable for analysis by the researcher. Data collected through a study is typically in its raw form. This form of data often has errors and inconsistencies which are not relevant to the study. Editing, coding, and tabulating are essential steps for the researcher to transform this raw data into a relevant dataset. This stage is important for effective research because processing the data helps reduce errors and bias, resulting in relevant and specific data that is suitable for analysis.
Data processing is the primary stage of data analysis. Setting up the collected data in a suitable format allows for computer coding and analysis. Data processing is essential for both evaluation and effective communication.
While processing the data, the researcher simplifies, conceptualises, and transforms the selected data into a proper form as per the research objectives. The refinement of data continues throughout the research process.
To maintain the integrity of the original data and achieve accurate analytical results, process the data carefully.
Preliminary preparation is necessary for the raw data from the questionnaire before applying statistical techniques for analysis. The quality of results obtained from statistical techniques and their interpretation heavily relies on the thorough preparation and conversion of data into an analyzable format.
Importance of Data Processing
Data processing provides the following advantages:
- Helps in decision making
- Provides Comprehensive Data
- Converts in a Suitable Format
- Provides Accurate Data
- Offers thorough data
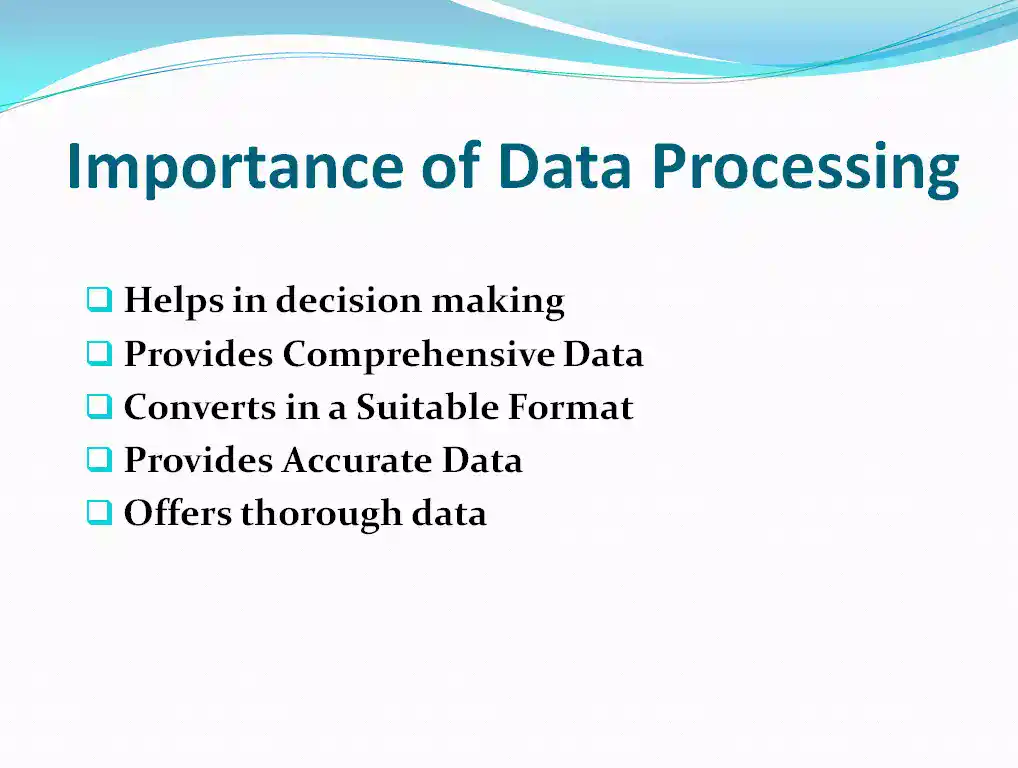
Data processing advantages are explained as follows:
1) Helps in Decision-Making: Processing the data also helps in decision making. Data processing operations transform the raw data into relevant information, which helps the managers make important decisions and take necessary actions.
2) Provides Comprehensive Data: Data processing makes the raw data concise, easy to read, and presentable. Managers can more easily understand processed data, saving time in the process.
3) Converts in a Suitable Format: Data processing enhances data efficiency by converting it into a computer-readable and comprehensive format, enabling quicker analysis.
4) Provides Accurate Data: In data processing, a researcher checks the accuracy of the data. Proper data processing eliminates all chances of errors in the collected data. Additionally, it guarantees data quality by correcting errors and omissions during the processing phase.
5) Offers thorough data: Data processing transforms raw data into meaningful information, enhancing the researcher’s understanding and enabling efficient analysis.
Data Analysis Meaning
Raw data in marketing research becomes usable only after undergoing processing. So, the conversion of raw data into information is necessary. In statistics, the basic arithmetic tools are ratios and percentiles.
Researchers employ various tools for data analysis, including mean, standard deviation, mode, median, coefficient of correlation, factor analysis, ANOVA, significance tests, cluster analysis, regression analysis, and multiple discriminant analyses as needed for their specific research goals.
Data analysis is the process of collecting, modelling and transforming data into valuable information that reveals outcomes and aids in decision making.
Related Articles:
- nature of marketing
- difference between questionnaire and schedule
- features of marginal costing
- placement in hrm
- limitations of marginal costing
- nature of leadership
- difference between advertising and personal selling
Phases of the Data Analysis
It’s possible to separate the data analysis process into two steps, as described.
1) Preliminary Data Analysis: Researchers perform preliminary data analysis to understand the various characteristics and attributes of the collected data. Researchers commonly undertake this analysis before conducting hypothesis tests or assessing data quality.
Researchers perform preliminary data analysis to assess the quality of data input, coding, and scale application before measuring it. This analysis also informs about the content of validity and the level of biasness. Knowledge gained during this phase affects the decisions and results of data analysis. By examining the result of various statistical tools such as central tendency, correlation, frequency distribution etc., preliminary data analysis is conducted.
2) Hypothesis Testing: Researchers before testing the hypothesis usually study the assumptions of the various statistical tests.
For well analysis of the results of tests, researchers study the assumption of the various statistical tests before performing the hypothesis testing. Hypothesis testing helps the researchers to make decisions using sampled data of an unknown distribution of a population and its parameters. It also determines the validity of various assumptions (i.e., null hypothesis) and states the selection and rejection of those assumptions.
Objectives of Data Analysis
1) Evaluate and enhance the quality of data.
2) Conduct a comparison between the target population and the population under study.
3) Predict the areas of potential errors (like non-response level, number of refusals attrition or the comparison groups).
4) Evaluate the measure of frequency and extent (means, medians etc.).
5) Evaluate the strength of association among variables.
6) Measure the level of uncertainty.
7) Observe and control the effects of other related features.
8) To make conclusions and make decisions.
9) To gain insight into the relationships that are observed or left unattended.
Types of Data Analysis
1) Descriptive and Causal Analysis: The descriptive analysis is used to summarize the data (collected from the population) which was observed in the sample, numerically or graphically.
According to Vogt, in descriptive statistics, we measure the central tendency, mean, mode, median, range, variance and standard deviation. This statistic summarizes the large data with one number and describes the characteristics of a relationship using diagrammatic and tabular methods.
2) Associational Statistics: It helps to calculate the significant relationships among electoral information dissemination variables such as radio, TV, print, personal contact and direct mail.
The following measures are used to calculate the significant relationship among variables:
i) Correlation Coefficient, and
ii) Multiple Regression Analysis
3) Inferential Analysis/Statistical Inference: The generalization of the results (taken through a random (probability) sample from the population) is done with the help of Inferential Analysis. The researchers use inferential analysis when they have collected the data from the sample by the random procedure with a high response rate. So, this method is not recommended when data collection is done via non-probability techniques with a low response rate.